Data science bootcamps are intensive short-term educational programs designed to equip individuals with the skills needed to enter or advance in the field of data science. They cover a wide range of topics, ranging from Python, R, and statistics to machine learning and data visualization.
These bootcamps are focused training and learning platforms for people. Nowadays, individuals tend to opt for bootcamps for quick results and faster learning of any particular niche.
In this blog, we will explore the arena of data science bootcamps and lay down a guide for you to choose the best data science bootcamp.
What do Data Science Bootcamps Offer?
Data science bootcamps offer a range of benefits designed to equip participants with the necessary skills to enter or advance in the field of data science. Here’s an overview of what these bootcamps typically provide:
Curriculum and Skills Learned
These bootcamps are designed to focus on practical skills and a diverse range of topics. Here’s a list of key skills that are typically covered in a good data science bootcamp:
- Programming Languages:
- Python: Widely used for its simplicity and extensive libraries for data analysis and machine learning.
- R: Often used for statistical analysis and data visualization.
- Data Visualization:
- Techniques and tools to create visual representations of data to communicate insights effectively. Tools like Tableau, Power BI, and Python libraries such as Matplotlib and Seaborn are commonly taught.
- Machine Learning:
- Supervised and unsupervised learning algorithms, including regression, classification, clustering, and deep learning. Tools and frameworks like Scikit-Learn, TensorFlow, and Keras are often covered.
- Big Data Technologies:
- Handling and processing large datasets using tools like Hadoop, Spark, and cloud platforms such as AWS and Google Cloud.
- Data Processing and Analysis:
- Techniques for data cleaning, manipulation, and analysis using libraries such as Pandas and Numpy in Python.
- Databases and SQL:
- Managing and querying relational databases using SQL, as well as working with NoSQL databases like MongoDB.
- Statistics:
- Fundamental statistical concepts and methods, including hypothesis testing, probability, and descriptive statistics.
- Data Engineering:
- Building and maintaining data pipelines, ETL (Extract, Transform, Load) processes, and data warehousing.
- Artificial Intelligence:
- Concepts of AI include neural networks, natural language processing (NLP), and reinforcement learning.
- Cloud Computing:
- Utilizing cloud services for data storage and processing, often covering platforms such as AWS, Azure, and Google Cloud.
- Soft Skills:
- Problem-solving, critical thinking, and communication skills to effectively work within a team and present findings to stakeholders.
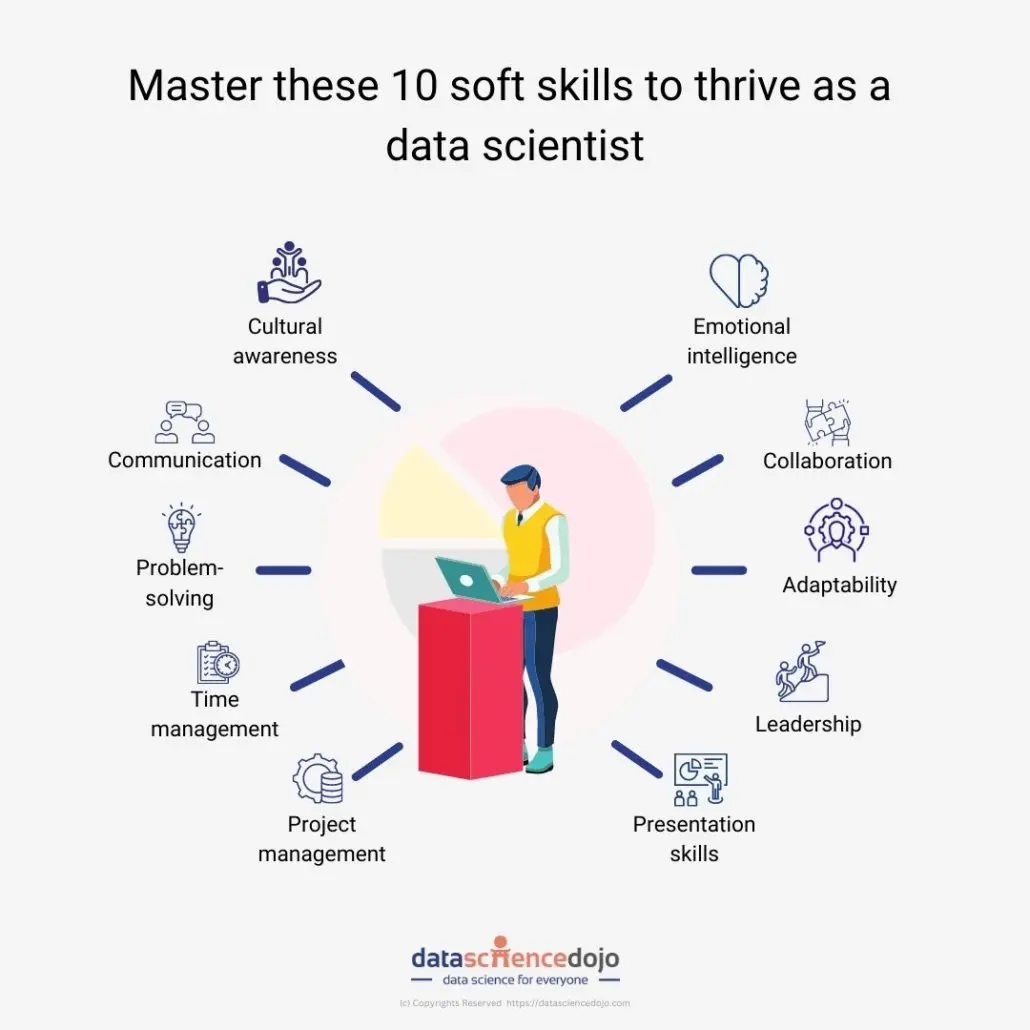
Moreover, these bootcamps also focus on hands-on projects that simulate real-world data challenges, providing participants a chance to integrate all the skills learned and assist in building a professional portfolio.
Learn more about key concepts of applied data science
Format and Flexibility
The bootcamp format is designed to offer a flexible learning environment. Today, there are bootcamps available in three learning modes: online, in-person, or hybrid. Each aims to provide flexibility to suit different schedules and learning preferences.
Career Support
Some bootcamps include job placement services like resume assistance, mock interviews, networking events, and partnerships with employers to aid in job placement. Participants often also receive one-on-one career coaching and support throughout the program.
Networking Opportunities
The popularity of bootcamps has attracted a diverse audience, including aspiring data scientists and professionals transitioning into data science roles. This provides participants with valuable networking opportunities and mentorship from industry professionals.
Admission and Prerequisites
Unlike formal degree programs, data science bootcamps are open to a wide range of participants, often requiring only basic knowledge of programming and mathematics. Some even offer prep courses to help participants get up to speed before the main program begins.
Real-World Relevance
The targeted approach of data science bootcamps ensures that the curriculum remains relevant to the advancements and changes of the real world. They are constantly updated to teach the latest data science tools and technologies that employers are looking for, ensuring participants learn industry-relevant skills.
Explore 6 ways to leverage LLMs as Data Scientists
Certifications
Certifications are another benefit of bootcamps. Upon completion, participants receive a certificate of completion or professional certification, which can enhance their resumes and career prospects.
Hence, data science bootcamps offer an intensive, practical, and flexible pathway to gaining the skills needed for a career in data science, with strong career support and networking opportunities built into the programs.
Factors to Consider when Choosing a Data Science Bootcamp
When choosing a data science bootcamp, several factors should be taken into account to ensure that the program aligns with your career goals, learning style, and budget.
Here are the key considerations to ensure you choose the best data science bootcamp for your learning and progress.
1. Outline Your Career Goals
A clear idea of what you want to achieve is crucial before you search for a data science bootcamp. You must determine your career objectives to ensure the bootcamp matches your professional interests. It also includes having the knowledge of specific skills required for your desired career path.
2. Research Job Requirements
As you identify your career goals, also spend some time researching the common technical and workplace skills needed for data science roles, such as Python, SQL, databases, machine learning, and data visualization. Looking at job postings is a good place to start your research and determine the in-demand skills and qualifications.
3. Assess Your Current Skills
While you map out your goals, it is also important to understand your current learning. Evaluate your existing knowledge and skills in data science to determine your readiness for a bootcamp. If you need to build foundational skills, consider beginner-friendly bootcamps or preparatory courses.
4. Research Programs
Once you have spent some time on the three steps above, you are ready to search for data science bootcamps. Some key factors for initial sorting include program duration, cost of the bootcamp, and the curriculum content. Consider what class structure and duration work best for your schedule and budget, and offer relevant course content.
5. Consider Structure and Location
With in-person, online, and hybrid formats, there are multiple options for you to choose from. Each format has its benefits, such as flexibility for online courses or hands-on experience in in-person classes. Consider your schedule and budget as you opt for a structure and format for your data science bootcamp.
6. Take Note of Relevant Topics
Some bootcamps offer specialized tracks or elective courses that align with specific career goals, such as machine learning or data engineering. Ensure that the bootcamp of your choice covers these specific topics. Moreover, you can confidently consider bootcamps that cover core topics like Python, machine learning, and statistics.
7. Know the Cost
Explore the financial requirements of the bootcamp you choose in detail. There can be some financial aid options available that you can benefit from. Other options to look for include scholarships, deferred tuition, income share agreements, or employer reimbursement programs to help offset the cost.
8. Research Institution Reputation
While course content and other factors are important, it is also crucial to choose from well-reputed options. Bootcamps from reputable institutions are a good place to look for such options. You can also read reviews from students and alumni to get a better idea of the options you are considering.
The quality of the bootcamp can also be measured through factors like instructor qualifications and industry partnerships. Moreover, also consider factors like career support services and the institution’s commitment to student success.
9. Analyze and Apply
This is the final step towards enrolling in a data science bootcamp. Weight the benefits of each option on your list against any potential drawbacks. After careful analysis, choose a bootcamp that meets your criteria. Complete their application form, and open up a world of learning and experimenting with data science.
From the above process and guidelines, it can be easily said that choosing the right data science bootcamp requires thorough research and consideration of various factors. By following a proper guideline, you can make an informed decision that aligns with your professional aspirations.
Comparing Different Options
The discussion around data science bootcamps also caters to multiple comparisons. The leading differences are drawn and analyzed to compare degree programs and bootcamps, and differentiate between in-person and online bootcamps.
Degree Programs vs Bootcamps
Both data science bootcamps and degree programs have distinct advantages and drawbacks. Bootcamps are ideal for those who want to quickly gain practical skills and enter the job market, while degree programs offer a more comprehensive and in-depth education.
Here’s a detailed comparison between both options for you.
Aspect | Data Science Degree Program | Data Science Bootcamp |
---|---|---|
Cost | Average in-state tuition: $53,100 | Typically costs between $7,500 and $27,500 |
Duration | Bachelor’s: 4 years; Master’s: 1-2 years | 3 to 6 months |
Skills Learned | Balance of theoretical and practical skills, including algorithms, statistics, and computer science fundamentals | Focus on practical, applied skills such as Python, SQL, machine learning, and data visualization |
Structure | Usually in-person; some universities offer online or hybrid options | Online, in-person, or hybrid models available |
Certification Type | Bachelor’s or Master’s degree | Certificate of completion or professional certification |
Career Support | Varies; includes career services departments, internships, and co-op programs | Extensive career services such as resume assistance, mock interviews, networking events, and job placement guarantees |
Networking Opportunities | Campus events, alumni networks, industry partnerships | Strong connections with industry professionals and companies, diverse participant background |
Flexibility | Less flexible; requires a full-time commitment | Offers flexible learning options including part-time and self-paced formats |
Long-Term Value | Provides a comprehensive education with a solid foundation for long-term career growth | Rapid skill acquisition for quick entry into the job market, but may lack depth |
While each option has its pros and cons, your choice should align with your career goals, current skill level, learning style, and financial situation.
Here’s a list of 10 best data science bootcamps
In-Person vs Online vs Hybrid Bootcamps
If you have decided to opt for a data science bootcamp to hone your skills and understanding, there are three different variations for you to choose from. Below is an overall comparison of all three approaches as you choose the most appropriate one for your learning.
Aspect | In-Person Bootcamps | Online Bootcamps | Hybrid Bootcamps |
---|---|---|---|
Learning Environment | A structured, hands-on environment with direct instructor interaction | Flexible, can be completed from anywhere with internet access | Combines structured in-person sessions with the flexibility of online learning |
Networking Opportunities | High, with opportunities for face-to-face networking and team-building | Lower compared to in-person, but can still include virtual networking events | Offers both in-person and virtual networking opportunities |
Flexibility | Less flexible, requires attendance at a physical location | Highly flexible, can be done at one’s own pace and schedule | Moderately flexible, includes both scheduled in-person and flexible online sessions |
Cost | Can be higher due to additional facility costs | Generally lower, no facility costs | Varies, but may involve some additional costs for in-person components |
Accessibility | Limited by geographical location, may require relocation or commute | Accessible to anyone with an internet connection and no geographical constraints | Accessible with some geographical constraints for the in-person part |
Interaction with Instructors | High, with immediate feedback and support | Can vary; some programs offer live support, others are more self-directed | High during in-person sessions, moderate online |
Learning Style Suitability | Best for those who thrive in a structured, interactive learning environment | Ideal for self-paced learners and those with busy schedules | Suitable for learners who need a balance of structure and flexibility |
Technical Requirements | Typically includes access to on-site resources and equipment | Requires a personal computer and reliable internet connection | Requires both access to a personal computer and traveling to a physical location |
Each type of bootcamp has its unique advantages and drawbacks. It is up to you to choose the one that aligns best with your learning practices.
What is the Future of Data Science Bootcamps?
The future of data science bootcamps looks promising, driven by several key factors that cater to the growing demand for data science skills in various industries.
One major factor is the increasing demand for skilled data scientists as companies across various industries harness the power of data to drive decision-making. The U.S. Bureau of Labor Statistics estimates the data science job outlook to be 35% between 2022–32, far above the average for all jobs of 2%.
Moreover, as the data science field evolves, bootcamps are likely to continue adapting their curriculum to incorporate emerging technologies and methodologies, such as artificial intelligence, machine learning, and big data analytics. It will continue to make them a favorable choice in this fast-paced digital world.
Hence, data science bootcamps are well-positioned to meet the increasing demand for data science skills. Their advantages in focused learning, practical experience, and flexibility make them an attractive option for a diverse audience. However, you should carefully evaluate bootcamp options to choose a program that meets your career goals.
Want to know more about data science, LLM, and bootcamps?
Join our Discord community for regular updates!