Large Language Models Bootcamp
NEW
. 5 DAYS . 40 HOURS .
IN-PERSON
Comprehensive, hands-on curriculum to give you a headstart in building Large Language Model Applications.
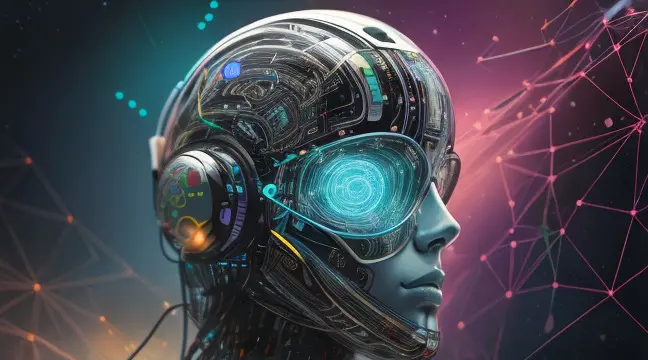
NEW
. 5 DAYS . 40 HOURS .
IN-PERSON
Software subscriptions and cloud services credit up to USD $500.
Breakfast, lunch and beverages daily.
Course material and access to coding labs, Jupyter notebooks, and hundreds of learning resources for 1 year.
INSTRUCTORS AND GUEST SPEAKERS
COURSE OVERVIEW
Pre-trained Large Language Models like ChatGPT offer impressive capabilities but they cannot be used in scenarios where the underlying data is proprietary and requires industry-specific knowledge. Businesses are rushing to build custom LLM applications that offer enhanced performance, control, customization and most importantly, competitive advantage.
This bootcamp offers a comprehensive introduction to get started with building a ChatGPT on your own data. By the end of the bootcamp, you will be capable of building LLM-powered applications on any dataset of your choice.
In collaboration with
Anyone interested in getting a headstart by getting a hands-on experience with building LLM applications.
Data professionals who want to supercharge their data skills using cutting-edge generative AI tools and techniques.
Product leaders at enterprises or startups seeking to leverage LLMs to enhance their products, processes and services.
A comprehensive introduction to the fundamentals of generative AI, foundation models and Large language models
An in-depth understanding of various LLM-powered application architectures and their relative tradeoffs
Hands-on experience with vector databases and vector embeddings
Practical experience with writing effective prompts for your LLM applications
Practical experience with orchestration frameworks like LangChain and Llama Index
Learn how to deploy your LLM applications using Azure and Hugging Face cloud
Practical experience with fine-tuning, parameter efficient tuning and retrieval parameter-efficient + retrieval-augmented approaches
A custom LLM application created on selected datasets
30% OFF
4.95 · 640+ reviews
Includes:
Software subscriptions and cloud services credit up to USD $500.
Breakfast, lunch and beverages daily.
Course material and access to coding labs, Jupyter notebooks, and hundreds of learning resources for 1 year.
Earn a Large Language Models certificate in association with the University of New Mexico Continuing Education, verifying your skills. Step into the market with a proven and trusted skillset.
In this module we will understand the common use cases of large language models and fundamental building blocks of such applications. Learners will be introduced to the following topics at a very high level without going into the technical details:
In this module, we will be reviewing how embeddings have evolved from the simplest one-hot encoding approach to more recent semantic embeddings approaches. The module will go over the following topics:
Hands-on Exercise
Dive into the world of large language models, discovering the potent mix of text embeddings, attention mechanisms, and the game-changing transformer model architecture.
Hands-on Exercise
Learn about efficient vector storage and retrieval with vector database, indexing techniques, retrieval methods, and hands-on exercises.
Understand how semantic search overcomes the fundamental limitation in lexical search i.e. lack of semantic . Learn how to use embeddings and similarity in order to build a semantic search model.
Unleash your creativity and efficiency with prompt engineering. Seamlessly prompt models, control outputs, and generate captivating content across various domains and tasks.
In-depth discussion on fine-tuning of large language models through theory discussions, exploring rationale, limitations, and Parameter Efficient Fine Tuning.
Explore the necessity of orchestration frameworks, tackling issues like foundation model retraining, token limits, data source connectivity, and boilerplate code. Discover popular frameworks, their creators, and open source availability.
Build LLM Apps using LangChain. Learn about LangChain's key components such as Models, Prompts, Parsers, Memory, Chains, and Question-Answering. Get hands-on evaluation experience.
Use LLMs to make decisions about what to do next. Enable these decisions with tools. In this module, we’ll talk about agents. We’ll learn what they are, how they work, and how to use them within the LangChain library to superpower our LLMs.
LLMOps encompasses the practices, techniques and tools used for the operational management of large language models in production environments. LLMs offer tremendous business value, humans are involved in all stages of the lifecycle of an LLM from acquisition of data to interpretation of insights. In this module we will learn about the following:
Dive into Large Language Model (LLM) evaluation, examining its importance, common issues, and key metrics such as BLEU and ROUGE, and apply these insights through a hands-on summarization exercise.
Introduction to LLM Evaluation Evaluation Metrics Hands-on Exercise
This module covers how to scale and automate LLM applications using ZenML. ZenML streamlines data versioning, caching, deployment, and collaboration for efficient LLM app development.
Key Challenges in building Enterprise-Level LLM Apps Hands-On Exercise
On the last day of the bootcamp, the learners will apply the concepts and techniques learned during the bootcamp to build an LLM application. Learners will choose to implement one of the following:
Attendees will receive the following: At the end of the bootcamp, you'll leave with a fully functional LLM application deployed in either Streamlit or Hugging Face cloud. Showcase your achievements and leverage the power of AI to transform your industry, gaining a competitive edge and staying ahead of the curve.
Daily schedule: 9 am - 5 pm | Breakfast, lunch and beverages | Breakout sessions and in-class activities
Yes, a very basic level of python programming language.
Yes.
You will leave the bootcamp with your own fully functional LLM application.
Yes, you can find out more here.
Data Science Dojo has conducted bootcamps in various cities in the past and plans to continue expanding to other locations. They are also exploring options for online bootcamps.
Yes, you will need to bring your laptop. As for software installation, we use browser-based coding labs. You will not need to install any software on your laptop.
Yes, you can fine-tune the LLM model on your own custom data sources. The bootcamp will provide guidance on how to add custom data sources and fine-tune the model to answer specific questions related to those sources. However, please make sure to get the dataset reviewed before the bootcamp starts to avoid any last-minute inconveniences.
Yes, participants who successfully complete the bootcamp will receive a certificate of completion. This certificate can be a valuable addition to your professional portfolio and demonstrate your expertise in building large language model applications.
Yes, the participants will have access to the learning platform for 12 months after the bootcamp ends.
Yes, Data Science Dojo provides ongoing support and guidance to bootcamp participants even after the program ends. This includes access to a community of fellow participants and instructors who can help answer questions and provide further assistance.
Yes, our live instructor-led sessions are interactive. During these sessions, students are encouraged to ask questions, and our instructors respond without rushing. Additionally, discussions within the scope of the topic being taught are actively encouraged. We understand that questions may arise during homework, and to assist with that, we offer office hours to help unblock students between sessions. Rest assured, you won't have to figure everything out by yourself – we are committed to providing the support you need for a successful learning experience.
Yes, a very basic level of python programming language.
Yes.
You will leave the bootcamp with your own fully functional LLM application.
Yes, you can find out more here.
Data Science Dojo has conducted bootcamps in various cities in the past and plans to continue expanding to other locations. They are also exploring options for online bootcamps.
Yes, you will need to bring your laptop. As for software installation, we use browser-based coding labs. You will not need to install any software on your laptop.
Yes, you can fine-tune the LLM model on your own custom data sources. The bootcamp will provide guidance on how to add custom data sources and fine-tune the model to answer specific questions related to those sources. However, please make sure to get the dataset reviewed before the bootcamp starts to avoid any last-minute inconveniences.
Yes, participants who successfully complete the bootcamp will receive a certificate of completion. This certificate can be a valuable addition to your professional portfolio and demonstrate your expertise in building large language model applications.
Yes, the participants will have access to the learning platform for 12 months after the bootcamp ends.
Yes, Data Science Dojo provides ongoing support and guidance to bootcamp participants even after the program ends. This includes access to a community of fellow participants and instructors who can help answer questions and provide further assistance.
Yes, our live instructor-led sessions are interactive. During these sessions, students are encouraged to ask questions, and our instructors respond without rushing. Additionally, discussions within the scope of the topic being taught are actively encouraged. We understand that questions may arise during homework, and to assist with that, we offer office hours to help unblock students between sessions. Rest assured, you won’t have to figure everything out by yourself – we are committed to providing the support you need for a successful learning experience.
PRICE
UPCOMING
DATES
APPLICATION DEADLINE