As a beginner in data science, one of the hardest things is to land their first job and to build an impressive portfolio. We are all aware of the vicious cycle of not getting a job because of no experience, and no experience because of no job.
Most of us get stuck in this cycle either when we are starting our careers or when we are transitioning into another career. A career in data science is no different, but the question arises of how to break through this cycle and land your first job.
To answer this, Data Science Dojo collaborated with Avery Smith to conduct a webinar for every beginner in data science who is stepping into the real world. He discussed some useful tips to help data scientists build a data science portfolio.
Avery’s secret to breaking into the data science industry is through “Projects”, which you can create to show off your skills and knowledge in your next interview. In this session, Avery took us through the best practices for creating a project that makes you stand out and helps you land your dream job.
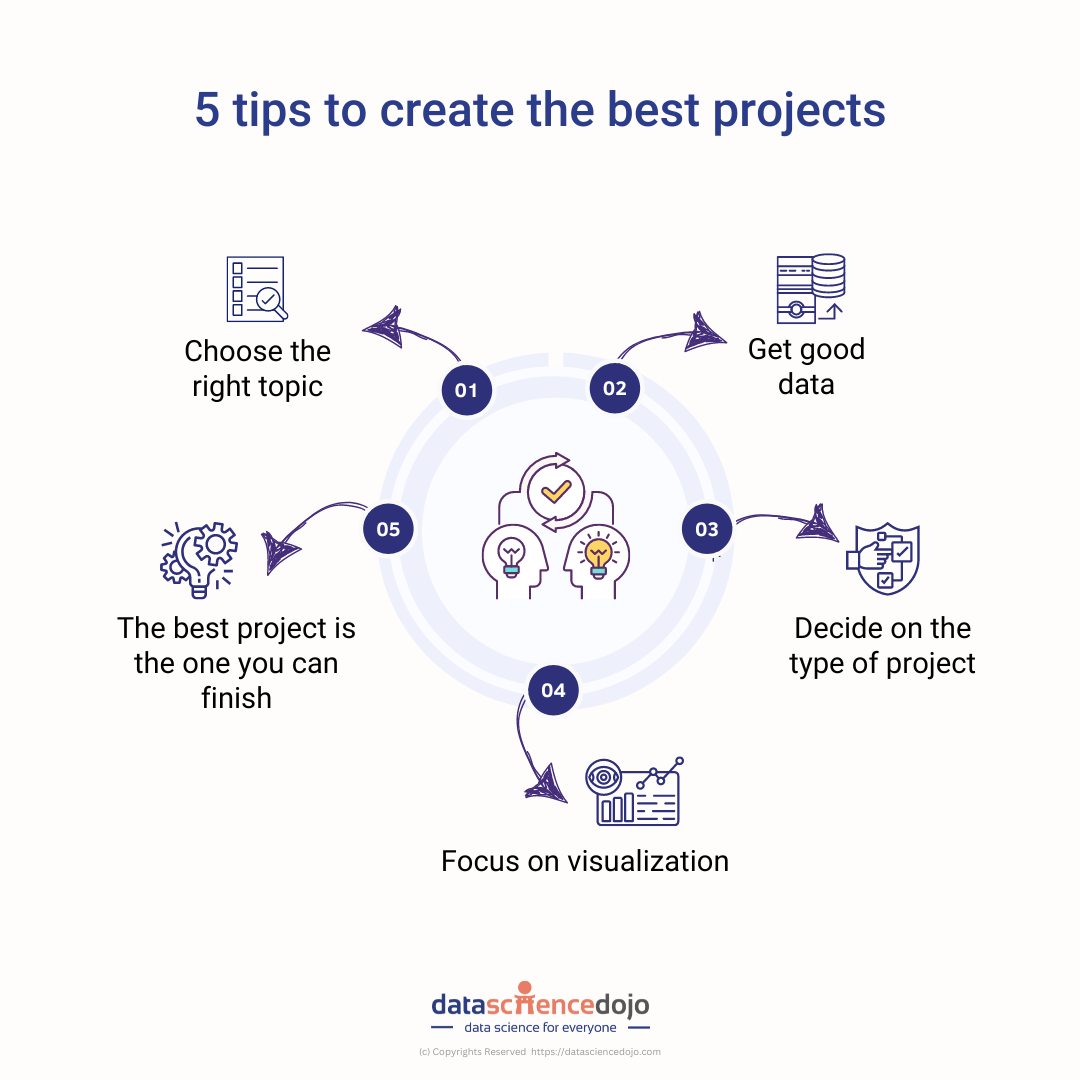
5 tips to create the best projects to improve portfolio
1. Choose the right topic
Choosing a topic that you can write passionately about is very important because that is the only way you will feel motivated to finish the project. If you are wondering where passion comes from, it could be something out of your hobbies or your next/dream job. The fun trick taught by Avery is to think about any hobby or industry you are passionate about.
Read more about data science portfolio
Next, go to your LinkedIn job section and search for data-related roles in the fields you are interested in. After that, find a job or company that you would like to work in, and scroll down to look for the qualifications required for that job.
For instance, if the job requires SQL, Python, and Tableau skills, you should create a project that involves these three. You will also look at what the company does and its job requirements, to make your project as relevant as possible.
2. Get good data
If you have successfully decided on a topic to work on, now you must be thinking about where to find relevant data. There are four main ways of gathering data, as Avery pointed out:
- Download CSV
- Using an API
- Web scraping
- Collecting your data
These four ways are mentioned in order to increase the difficulty to get each and the more unique it is. Although downloading a CSV is easy, it’s not overly impressive. Collecting your own data is exceedingly difficult but is unique and will make a larger impact in showing off your skillset.
3. Decide on the type of project
There are three types of projects:
- Skillshare- a few steps in Python or a SQL query or a graph in the dashboard. It’s not like a whole project but a section of the project.
- Data Story- a whole paragraph with multiple lines of codes, and multiple graphs which is more like a complete article.
- Product- a tool or app that you can give to someone, and they can use it.
The types are in order of increasing difficulty and impressiveness, skill share is easiest to do but not very impressive while on the other hand product is very difficult but highly impressive. In the webinar, Avery explained these using examples for each type of project.
4. Focus on visualization
Visualization is one of the easiest to do, looks impressive, and you can start it today. For beginners who feel like they are not ready to work on a big project, data visualization is something you can start working on day one. There are several tools and software available which are easy to learn and can help in creating amazing projects, you can learn more about visualization tips and techniques.
5. The best project is the one you can finish
Many data scientists have several projects that they started but never got the chance to finish. A very little-known fact is that these projects can become their marketeers by attracting recruiters and helping them land the right job. For that you need to get these projects out there, nothing is going to happen if keep them restricted to your computer.
For this reason, we need to finish and publish these projects. Avery’s advice has been to avoid the scenario where you have several unfinished projects and you decide to start another, the goal is to have published projects. To better understand it, Avery introduced us to the concept of Modular Projects.
What are modular projects
Avery explained the concept of modular projects with marathons. People who run a marathon don’t do it all at once. First, they run 5k, then 10k, maybe a half marathon, and probably then they can run a full marathon. Similarly for a project, don’t go for a marathon project off the start. Instead, start with 5k.
You can always imagine a marathon, but try to reach a 5k first, publish, and then move ahead for a 10k. The idea of a modular project is to pick a low finish line and work your way up.
In nutshell, Avery provided all beginners with a starting point to enter their careers and prove themselves. This is your sign to start building a project right away, considering all the tips and tricks given in the webinar.