Traditional databases in healthcare struggle to grasp the complex relationships between patients and their clinical histories. This limitation hinders personalized medicine and hampers rapid diagnosis. Vector databases, with their ability to store and query high-dimensional patient data, emerge as a revolutionary solution.
This blog delves into the technical details of how AI in healthcare empowers patient similarity searches and paves the path for precision medicine.
Impact of AI on healthcare
The healthcare landscape is brimming with data such as demographics, medical records, lab results, imaging scans, – the list goes on. While these large datasets hold immense potential for personalized medicine and groundbreaking discoveries, traditional relational databases cannot store such high-dimensional data at a large scale and often fall short.
Their rigid structure struggles to represent the intricate connections and nuances inherent in patient data.
Vector databases are revolutionizing healthcare data management. Unlike traditional, table-like structures, they excel at handling the intricate, multi-dimensional nature of patient information.
Each patient becomes a unique point in a high-dimensional space, defined by their genetic markers, lab values, and medical history. This dense representation unlocks powerful capabilities discussed later.
Working with vector data is tough because regular databases, which usually handle one piece of information at a time, can’t handle the complexity and large amount of this type of data. This makes it hard to find important information and analyze it quickly.
That’s where vector databases come in handy—they are made on purpose to handle this special kind of data. They give you the speed, ability to grow, and flexibility you need to get the most out of your data.
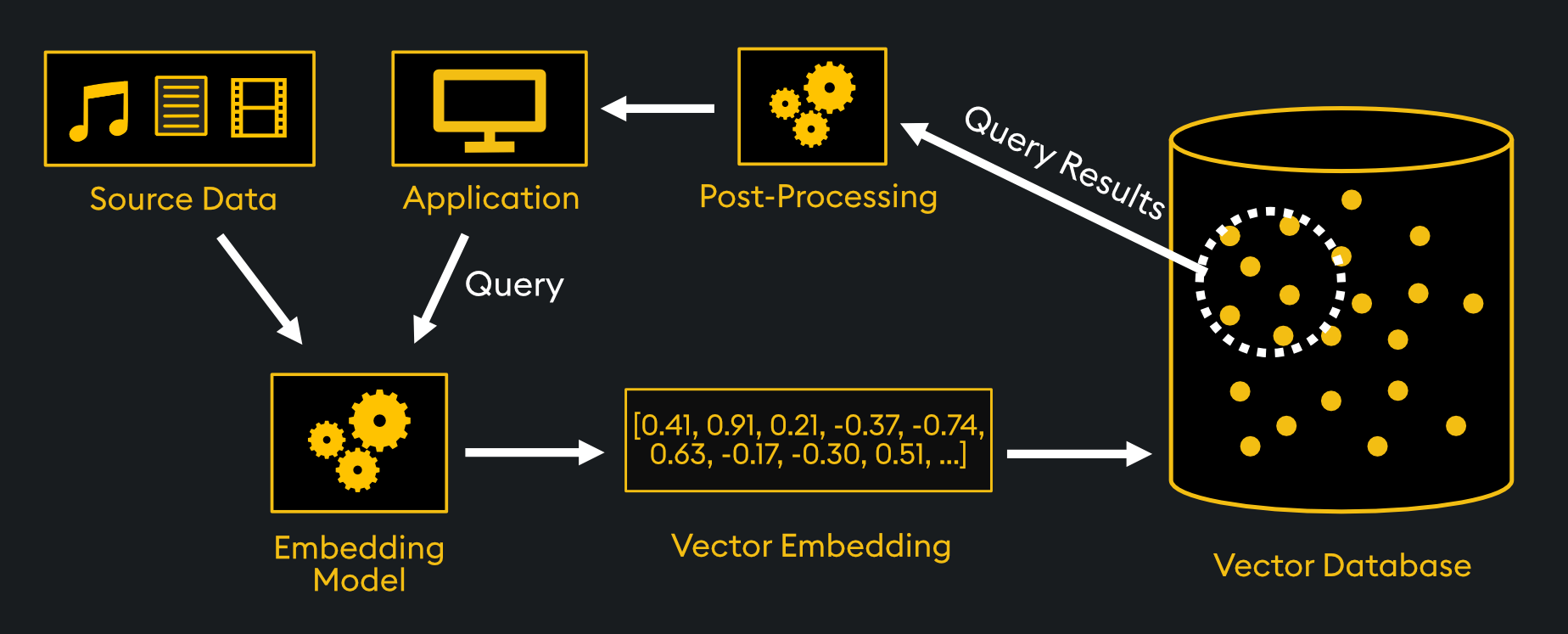
Patient similarity search with vector databases in healthcare
The magic lies in the ability to perform a similarity search. By calculating the distance between patient vectors, we can identify individuals with similar clinical profiles. This opens a large span of possibilities.
Personalized treatment plans
By uncovering patients with comparable profiles and treatment outcomes, doctors can tailor interventions with greater confidence and optimize individual care. It also serves as handy for medical researchers to look for efficient cures or preventions for a disease diagnosed over multiple patients by analyzing their data, particularly for a certain period.
Here’s how vector databases transform treatment plans:
- Precise Targeting: By comparing a patient’s vector to those of others who have responded well to specific treatments, doctors can identify the most promising options with laser-like accuracy. This reduces the guesswork and minimizes the risk of ineffective therapies.
- Predictive Insights: Vector databases enable researchers to analyze the trajectories of similar patients, predicting their potential responses to different treatments. This foresight empowers doctors to tailor interventions, preventing complications and optimizing outcomes proactively.
- Unlocking Untapped Potential: By uncovering hidden connections between seemingly disparate data points, vector databases can reveal new therapeutic targets and treatment possibilities. This opens doors for personalized medicine breakthroughs that were previously unimaginable.
- Dynamic Adaptation: As a patient’s health evolves, their vector map shifts and readjusts accordingly. This allows for real-time monitoring and continuous refinement of treatment plans, ensuring the best possible care at every stage of the journey.
Drug discovery and repurposing
Identifying patients similar to those successfully treated with a specific drug can accelerate clinical trials and uncover unexpected connections for existing medications.
- Accelerated exploration: They transform complex drug and disease data into dense vectors, allowing for rapid similarity searches and the identification of promising drug candidates. Imagine sifting through millions of molecules at a single glance, pinpointing those with similar properties to known effective drugs.
- Repurposing potential: Vector databases can unearth hidden connections between existing drugs and potential new applications. By comparing drug vectors to disease vectors, they can reveal unexpected repurposing opportunities, offering a faster and cheaper path to new treatments.
- Personalization insights: By weaving genetic and patient data into the drug discovery tapestry, vector databases can inform the development of personalized medications tailored to individual needs and responses. This opens the door to a future where treatments are as unique as the patients themselves.
- Predictive power: Analyzing the molecular dance within the vector space can unveil potential side effects and predict drug efficacy before entering clinical trials. This helps navigate the treacherous waters of development, saving time and resources while prioritizing promising candidates.
Cohort analysis in research
Grouping patients with similar characteristics facilitates targeted research efforts, leading to faster breakthroughs in disease understanding and treatment development.
- Exploring Disease Mechanisms: Vector databases facilitate the identification of patient clusters that share similar disease progression patterns. This can shed light on underlying disease mechanisms and guide the development of novel diagnostic markers and therapeutic target
- Unveiling Hidden Patterns: Vector databases excel at similarity search, enabling researchers to pinpoint patients with similar clinical trajectories, even if they don’t share the same diagnosis or traditional risk factors. This reveals hidden patterns that might have been overlooked in traditional data analysis methods.
Technicalities of vector databases
Using a vector database enables the incorporation of advanced functionalities into our artificial intelligence, such as semantic information retrieval and long-term memory. The diagram provided below enhances our comprehension of the significance of vector databases in such applications.
Let’s break down the illustrated process:
- Initially, we employ the embedding model to generate vector embeddings for the content intended for indexing.
- The resulting vector embedding is then placed into the vector database, referencing the original content from which the embedding was derived.
- Upon receiving a query from the application, we utilize the same embedding model to create embeddings for the query. These query embeddings are subsequently used to search the database for similar vector embeddings. As previously noted, these analogous embeddings are linked to the initial content from which they were created.
In comparison to the working of a traditional database, where data is stored as common data types like string, integer, date, etc. Users query the data by comparison with each row; the result of this query is the rows where the condition of the query is withheld.
In vector databases, this process of querying is more optimized and efficient with the use of a similarity metric for searching the most similar vector to our query. The search involves a combination of various algorithms, like approximate nearest neighbor optimization, which uses hashing, quantization, and graph-based detection.
Here are a few key components of the discussed process described below:
- Feature engineering: Transforming raw clinical data into meaningful numerical representations suitable for vector space. This may involve techniques like natural language processing for medical records or dimensionality reduction for complex biomolecular data.
- Distance metrics: Choosing the appropriate distance metric to calculate the similarity between patient vectors. Popular options include Euclidean distance, cosine similarity, and Manhattan distance, each capturing different aspects of the data relationships.
-
- Cosine Similarity: Calculates the cosine of the angle between two vectors in a vector space. It varies from -1 to 1, with 1 indicating identical vectors, 0 denoting orthogonal vectors, and -1 representing diametrically opposed vectors.
-
- Euclidean Distance: Measures the straight-line distance between two vectors in a vector space. It ranges from 0 to infinity, where 0 signifies identical vectors and larger values indicate increasing dissimilarity between vectors.
-
- Dot Product: Evaluate the product of the magnitudes of two vectors and the cosine of the angle between them. Its range is from -∞ to ∞, with a positive value indicating vectors pointing in the same direction, 0 representing orthogonal vectors, and a negative value signifying vectors pointing in opposite directions.
- Nearest neighbor search algorithms: Efficiently retrieving the closest patient vectors to a given query. Techniques like k-nearest neighbors (kNN) and Annoy trees excel in this area, enabling rapid identification of similar patients.
A general pipeline from storing vectors to querying them is shown in the figure below:
- Indexing: The vector database utilizes algorithms like PQ, LSH, or HNSW (detailed below) to index vectors. This process involves mapping vectors to a data structure that enhances search speed.
- Querying: The vector database examines the indexed query vector against the dataset’s indexed vectors, identifying the nearest neighbors based on a similarity metric employed by that specific index.
- Post Processing: In certain instances, the vector database retrieves the ultimate nearest neighbors from the dataset and undergoes post-processing to deliver the final results. This step may involve re-evaluating the nearest neighbors using an alternative similarity measure.
Challenges and considerations
While vector databases offer immense potential, challenges remain:
- Data privacy and security: Safeguarding patient data while harnessing its potential for enhanced healthcare outcomes requires the implementation of robust security protocols and careful consideration of ethical standards.
This involves establishing comprehensive measures to protect sensitive information, ensuring secure storage, and implementing stringent access controls.
Additionally, ethical considerations play a pivotal role, emphasizing the importance of transparent data handling practices, informed consent procedures, and adherence to privacy regulations. As healthcare organizations leverage the power of data to advance patient care, a meticulous approach to security and ethics becomes paramount to fostering trust and upholding the integrity of the healthcare ecosystem.
- Explainability and interpretability: Gaining insight into the reasons behind patient similarity is essential for informed clinical decision-making. It is crucial to develop transparent models that not only analyze the “why” behind these similarities but also offer insights into the importance of features within the vector space.
This transparency ensures a comprehensive understanding of the factors influencing patient similarities, contributing to more effective and reasoned clinical decisions. Integration with existing infrastructure: Seamless integration with legacy healthcare systems is essential for the practical adoption of vector database technology.
Revolution of medicine – AI in healthcare
In summary, the integration of vector databases in healthcare is revolutionizing patient care and diagnostics. Overcoming the limitations of traditional systems, these databases enable efficient handling of complex patient data, leading to precise treatment plans, accelerated drug discovery, and enhanced research capabilities.
While the technical aspects showcase the sophistication of these systems, challenges such as data privacy and seamless integration with existing infrastructure need attention. Despite these hurdles, the potential benefits promise a significant impact on personalized medicine and improved healthcare outcomes.