Will machines ever think, learn, and innovate like humans?
This bold question lies at the heart of Artificial General Intelligence (AGI), a concept that has fascinated scientists and technologists for decades.
Unlike the narrow AI systems we interact with today—like voice assistants or recommendation engines—AGI aims to replicate human cognitive abilities, enabling machines to understand, reason, and adapt across a multitude of tasks.
Current AI models, such as GPT-4, are gaining significant popularity due to their ability to generate outputs for various use cases without special prompting.
While they do exhibit early forms of what could be considered AGI, they are still far from achieving true AGI. Read more
But what is Artificial General Intelligence exactly, and how far are we from achieving it?
This article dives into the nuances of AGI, exploring its potential, current challenges, and the groundbreaking research propelling us toward this ambitious goal.
What is Artificial General Intelligence
Artificial General Intelligence is a theoretical form of artificial intelligence that aspires to replicate the full range of human cognitive abilities. AGI systems would not be limited to specific tasks or domains but would possess the capability to perform any intellectual task that a human can do. This includes understanding, reasoning, learning from experience, and adapting to new tasks without human intervention.
Qualifying AI as AGI
To qualify as AGI, an AI system must demonstrate several key characteristics that distinguish it from narrow AI applications:
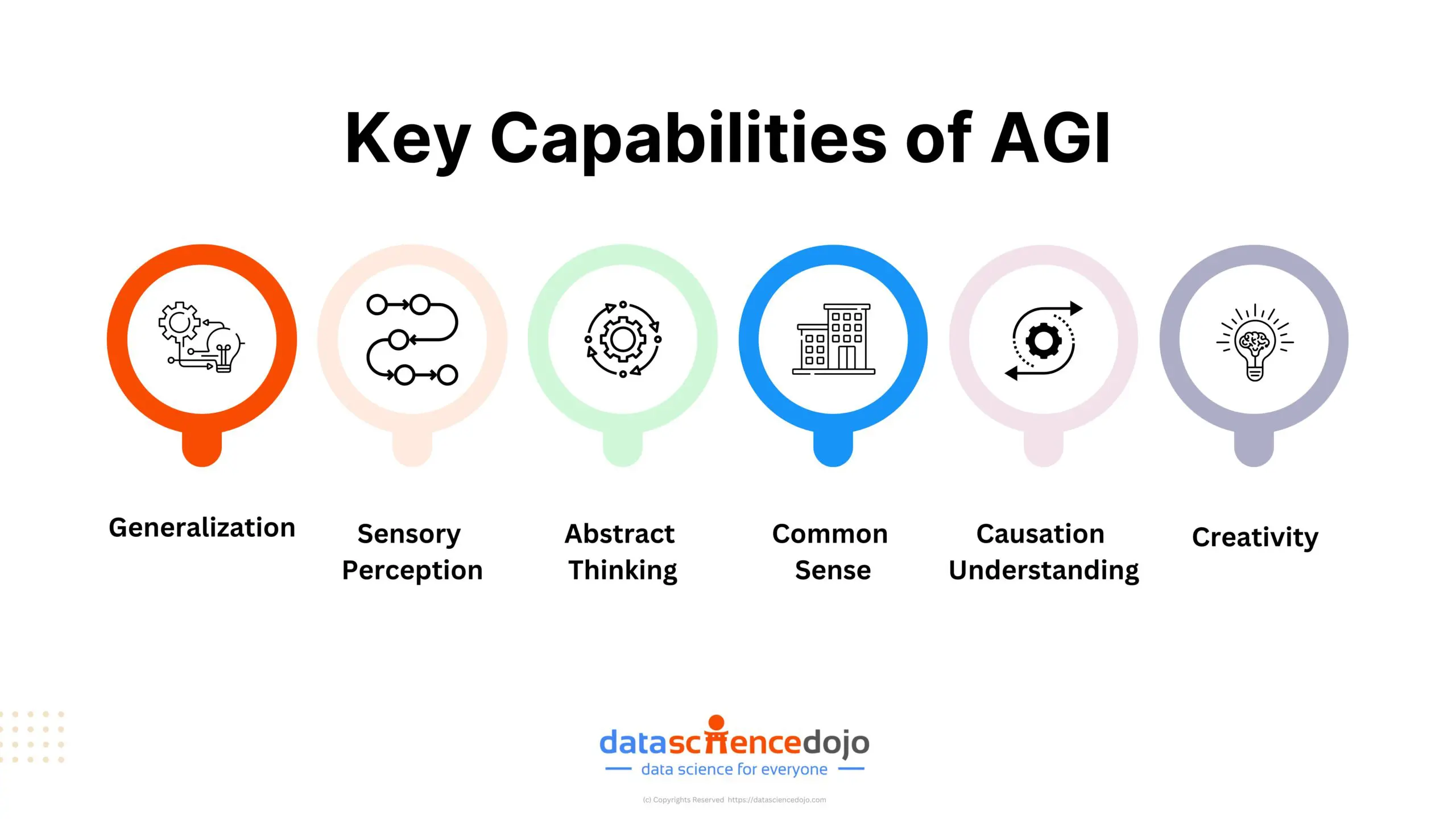
- Generalization Ability: AGI can transfer knowledge and skills learned in one domain to another, enabling it to adapt to new and unseen situations effectively.
- Common Sense Knowledge: Artificial General Intelligence possesses a vast repository of knowledge about the world, including facts, relationships, and social norms, allowing it to reason and make decisions based on this understanding.
- Abstract Thinking: The ability to think abstractly and infer deeper meanings from given data or situations.
- Causation Understanding: A thorough grasp of cause-and-effect relationships to predict outcomes and make informed decisions.
- Sensory Perception: Artificial General Intelligence systems would need to handle sensory inputs like humans, including recognizing colors, depth, and other sensory information.
- Creativity: The ability to create new ideas and solutions, not just mimic existing ones. For instance, instead of generating a Renaissance painting of a cat, AGI would conceptualize and paint several cats wearing the clothing styles of each ethnic group in China to represent diversity.
Current Research and Developments in Artificial General Intelligence
- Large Language Models (LLMs):
- GPT-4 is a notable example of recent advancements in AI. It exhibits more general intelligence than previous models and is capable of solving tasks in various domains such as mathematics, coding, medicine, and law without special prompting. Its performance is often close to a human level and surpasses prior models like ChatGPT.
-
- GPT-4’s capabilities are a significant step towards AGI, demonstrating its potential to handle a broad swath of tasks with human-like performance. However, it still has limitations, such as planning and real-time adaptability, which are essential for true AGI.
- Symbolic and Connectionist Approaches:
- Researchers are exploring various theoretical approaches to develop AGI, including symbolic AI, which uses logic networks to represent human thoughts, and connectionist AI, which replicates the human brain’s neural network architecture.
- The connectionist approach, often seen in large language models, aims to understand natural languages and demonstrate low-level cognitive capabilities.
- Hybrid Approaches:
- The hybrid approach combines symbolic and sub-symbolic methods to achieve results beyond a single approach. This involves integrating different principles and methods to develop AGI.
- Robotics and Embodied Cognition:
- Advanced robotics integrated with AI is pivotal for AGI development. Researchers are working on robots that can emulate human actions and movements using large behavior models (LBMs).
- Robotic systems are also crucial for introducing sensory perception and physical manipulation capabilities required for AGI systems 2.
- Computing Advancements:
- Significant advancements in computing infrastructure, such as Graphics Processing Units (GPUs) and quantum computing, are essential for AGI development. These technologies enable the processing of massive datasets and complex neural networks.
Pioneers in the Field of AGI
The field of AGI has been significantly shaped by both early visionaries and modern influencers.
Their combined efforts in theoretical research, practical applications, and ethical considerations continue to drive the field forward.
Understanding their contributions provides valuable insights into the ongoing quest to create machines with human-like cognitive abilities.
Early Visionaries
- John McCarthy, Marvin Minsky, Nat Rochester, and Claude Shannon:
- Contributions: These early pioneers organized the Dartmouth Conference in 1956, which is considered the birth of AI as a field. They conjectured that every aspect of learning and intelligence could, in principle, be so precisely described that a machine could be made to simulate it.
- Impact: Their work laid the groundwork for the conceptual framework of AI, including the ambitious goal of creating machines with human-like reasoning abilities.
2. Nils John Nilsson:
- Contributions: Nils John Nilsson was a co-founder of AI as a research field and proposed a test for human-level AI focused on employment capabilities, such as functioning as an accountant or a construction worker.
- Impact: His work emphasized the practical application of AI in varied domains, moving beyond theoretical constructs.
Modern Influencers
- Shane Legg and Demis Hassabis:
- Contributions: Co-founders of DeepMind have been instrumental in advancing the concept of AGI. DeepMind’s mission to “solve intelligence” reflects its commitment to creating machines with human-like cognitive abilities.
- Impact: Their work has resulted in significant milestones, such as the development of AlphaZero, which demonstrates advanced general-purpose learning capabilities.
2. Ben Goertzel:
- Contributions: Goertzel is known for coining the term “Artificial General Intelligence” and for his work on the OpenCog project, an open-source platform aimed at integrating various AI components to achieve AGI.
- Impact: He has been a vocal advocate for AGI and has contributed significantly to both the theoretical and practical aspects of the field.
3. Andrew Ng:
- contributions: While often critical of the hype surrounding AGI, Ng has organized workshops and contributed to discussions about human-level AI. He emphasizes the importance of solving real-world problems with current AI technologies while keeping an eye on the future of AGI.
- Impact: His balanced perspective helps manage expectations and directs focus toward practical AI applications.
4. Yoshua Bengio:
- Contributions: A co-winner of the Turing Award, Bengio has suggested that achieving AGI requires giving computers common sense and causal inference capabilities.
- Impact: His research has significantly influenced the development of deep learning and its applications in understanding human-like intelligence.
What is Stopping Us from Reaching AGI?
Achieving Artificial General Intelligence (AGI) involves complex challenges across various dimensions of technology, ethics, and resource management. Here’s a more detailed exploration of the obstacles:
- The complexity of Human Intelligence:
- Human cognition is incredibly complex and not entirely understood by neuroscientists or psychologists. AGI requires not only simulating basic cognitive functions but also integrating emotions, social interactions, and abstract reasoning, which are areas where current AI models are notably deficient.
- The variability and adaptability of human thought processes pose a challenge. Humans can learn from limited data and apply learned concepts in vastly different contexts, a flexibility that current AI lacks.
- Computational Resources:
- The computational power required to achieve general intelligence is immense. Training sophisticated AI models involves processing vast amounts of data, which can be prohibitive in terms of energy consumption and financial cost.
- The scalability of hardware and the efficiency of algorithms need significant advancements, especially for models that would need to operate continuously and process information from a myriad of sources in real time.
- Safety and Ethics:
- The development of such a technology raises profound ethical concerns, including the potential for misuse, privacy violations, and the displacement of jobs. Establishing effective regulations to mitigate these risks without stifling innovation is a complex balance to achieve.
- There are also safety concerns, such as ensuring that systems possessing such powers do not perform unintended actions with harmful consequences. Designing fail-safe mechanisms that can control highly intelligent systems is an ongoing area of research.
- Data Limitations:
- Artificial General Intelligence requires diverse, high-quality data to avoid biases and ensure generalizability. Most current datasets are narrow in scope and often contain biases that can lead AI systems to develop skewed understandings of the world.
- The problem of acquiring and processing the amount and type of data necessary for true general intelligence is non-trivial, involving issues of privacy, consent, and representation.
- Algorithmic Advances:
- Current algorithms primarily focus on specific domains (like image recognition or language processing) and are based on statistical learning approaches that may not be capable of achieving the broader understanding required for AGI.
- Innovations in algorithmic design are required that can integrate multiple types of learning and reasoning, including unsupervised learning, causal reasoning, and more.
- Scalability and Generalization:
- AI models today excel in controlled environments but struggle in unpredictable settings—a key feature of human intelligence. AGI requires a system to adapt new knowledge across various domains without extensive retraining.
- Developing algorithms that can generalize from few examples across diverse environments is a key research area, drawing from both deep learning and other forms of AI like symbolic AI.
- Integration of Multiple AI Systems:
- AGI would likely need to seamlessly integrate specialized systems such as natural language processors, visual recognizers, and decision-making models. This integration poses significant technical challenges, as these systems must not only function together but also inform and enhance each other’s performance.
- The orchestration of these complex systems to function as a cohesive unit without human oversight involves challenges in synchronization, data sharing, and decision hierarchies.
Each of these areas not only presents technical challenges but also requires consideration of broader impacts on society and individual lives. The pursuit of AGI thus involves multidisciplinary collaboration beyond the field of computer science, including ethics, philosophy, psychology, and public policy.
What is Artificial General Intelligence Future
The quest to understand if machines can truly think, learn, and innovate like humans continues to push the boundaries of Artificial General Intelligence. This pursuit is not just a technical challenge but a profound journey into the unknown territories of human cognition and machine capability.
Despite considerable advancements in AI, such as the development of increasingly sophisticated large language models like GPT-4, which showcase impressive adaptability and learning capabilities, we are still far from achieving true AGI. These models, while advanced, lack the inherent qualities of human intelligence such as common sense, abstract thinking, and a deep understanding of causality—attributes that are crucial for genuine intellectual equivalence with humans.
Thus, while the potential of AGI to revolutionize our world is immense—offering prospects that range from intelligent automation to deep scientific discoveries—the path to achieving such a technology is complex and uncertain. It requires sustained, interdisciplinary efforts that not only push forward the frontiers of technology but also responsibly address the profound implications such developments would have on society and human life.