Data science, machine learning, artificial intelligence, and statistics can be complex topics. But that doesn’t mean they can’t be fun! Memes and jokes are a great way to learn about these topics in a more light-hearted way.
In this blog, we’ll take a look at some of the best memes and jokes about data science, machine learning, artificial intelligence, and statistics. We’ll also discuss why these memes and jokes are so popular, and how they can help us learn about these topics.
So, whether you’re a data scientist, a machine learning engineer, or just someone who’s interested in these topics, read on for a laugh and a learning experience!
1. Data Science Memes
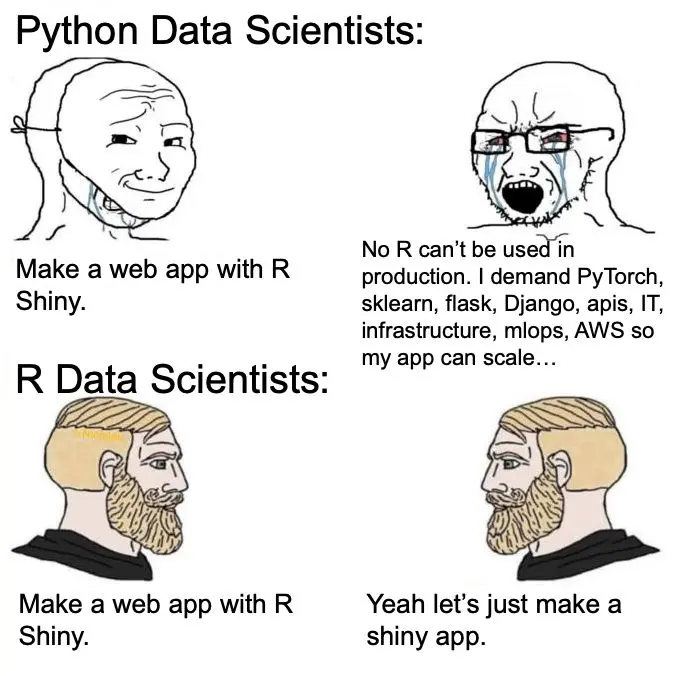
As a data scientist, you must be able to relate to the above meme. R is a popular language for statistical computing, while Python is a general-purpose language that is also widely used for data science. They both are the most used languages in data science having their own advantages.
Here is a more detailed explanation of the two languages:
- R is a statistical programming language that is specifically designed for data analysis and visualization. It is a powerful language with a wide range of libraries and packages, making it a popular choice for data scientists.
- Python is a general-purpose programming language that can be used for a variety of tasks, including data science. It is a relatively easy language to learn, and it has a large and active community of developers.
Both R and Python are powerful languages that can be used for data science. The best language for you will depend on your specific needs and preferences. If you are looking for a language that is specifically designed for statistical computing, then R is a good choice. If you are looking for a language that is more versatile and can be used for a variety of tasks, then Python is a good choice.
Here are some additional thoughts on R and Python in data science:
- R is often seen as the better language for statistical analysis, while Python is often seen as the better language for machine learning. However, both languages can be used for both tasks.
- R is generally slower than Python, but it is more expressive and has a wider range of libraries and packages.
- Python is easier to learn than R, but it has a steeper learning curve for statistical analysis.
Ultimately, the best language for you will depend on your specific needs and preferences. If you are not sure which language to choose, I recommend trying both and seeing which one you prefer.
We’ve been on Twitter for a while now and noticed that there’s always a new tool or app being announced. It’s like the world of tech is constantly evolving, and we’re all just trying to keep up.
Although we are constantly learning about new tools and looking for ways to improve the workflow. But sometimes, it can be a bit overwhelming. There’s just so much information out there, and it’s hard to know which tools are worth your time.
So, what should we do to efficiently learn about evolving technology? We can develop a bit of a filter when it comes to new tools. If you see a tweet about a new tool, first ask yourself: “What problem does this tool solve?” If the answer is something that I’m currently struggling with, then take a closer look.
Also, check out the reviews for the tool. If the reviews are mostly positive, then try it. But if the reviews are mixed, then you can probably pass. Just
Just remember to be selective about the tools you use. Don’t just install every new tool that you see. Instead, focus on the tools that will actually help you be more productive.
And who knows, maybe you’ll even be the one to announce the next big thing!
Enjoying this blog? Read more about —> Data Science Jokes
2. Machine Learning Meme
Despite these challenges, machine learning is a powerful tool that can be used to solve a wide range of problems. However, it is important to be aware of the potential for confusion when working with machine learning.
Here are some tips for dealing with confusing machine learning:
- Find a good resource. There are many good resources available that can help you understand machine learning. These resources can include books, articles, tutorials, and online courses.
- Don’t be afraid to ask for help. If you are struggling to understand something, don’t be afraid to ask for help from a friend, colleague, or online forum.
- Take it slow. Machine learning is a complex field, and it takes time to learn. Don’t try to learn everything at once. Instead, focus on one concept at a time and take your time.
- Practice makes perfect. The best way to learn machine learning is by practicing. Try to build your own machine-learning models and see how they perform.
With time and effort, you can overcome the confusion and learn to use machine learning to solve real-world problems.
3. Statistics Meme
Here are some fun examples to understand about outliers in linear regression models:
Outliers are like weird kids in school. They don’t fit in with the rest of the data, and they can make the model look really strange.
Outliers are like bad apples in a barrel. They can spoil the whole batch, and they can make the model inaccurate.
Outliers are like the drunk guy at a party. They’re not really sure what they’re doing, and they’re making a mess.
So, how do you deal with outliers in linear regression models? There are a few things you can do:
- You can try to identify the outliers and remove them from the data set. This is a good option if the outliers are clearly not representative of the overall trend.
- You can try to fit a non-linear regression model to the data. This is a good option if the data does not follow a linear trend.
- You can try to adjust the model to account for the outliers. This is a more complex option, but it can be effective in some cases.
Ultimately, the best way to deal with outliers in linear regression models depends on the specific data set and the goals of the analysis.
4. Programming Language Meme
Java and Python are two of the most popular programming languages in the world. They are both object-oriented languages, but they have different syntax and semantics.
Here is a simple code written in Java:
And here is the same code written in Python:
As you can see, the Java code is more verbose than the Python code. This is because Java is a statically typed language, which means that the types of variables and expressions must be declared explicitly. Python, on the other hand, is a dynamically typed language, which means that the types of variables and expressions are inferred by the interpreter.
The Java code is also more structured than the Python code. This is because Java is a block-structured language, which means that statements must be enclosed in blocks. Python, on the other hand, is a free-form language, which means that statements can be placed anywhere on a line.
So, which language is better? It depends on your needs. If you need a language that is statically typed and structured, then Java is a good choice. If you need a language that is dynamically typed and free-form, then Python is a good choice.
Here is a light and funny way to think about the difference between Java and Python:
- Java is like a suit and tie. It’s formal and professional.
- Python is like a T-shirt and jeans. It’s casual and relaxed.
- Java is like a German car. It’s efficient and reliable.
- Python is like a Japanese car. It’s fun and quirky.
Ultimately, the best language for you depends on your personal preferences. If you’re not sure which language to choose, I recommend trying both and seeing which one you like better.
Git pull and git push are two of the most common commands used in Git. They are used to synchronize your local repository with a remote repository.
Git pull fetches the latest changes from the remote repository and merges them into your local repository.
Git push pushes your local changes to the remote repository.
Here is a light and funny way to think about git pull and git push:
- Git pull is like asking your friend to bring you a beer. You’re getting something that’s already been made, and you’re not really doing anything.
- Git push is like making your own beer. It’s more work, but you get to enjoy the fruits of your labor.
- Git pull is like a lazy river. You just float along and let the current take you.
- Git push is like whitewater rafting. It’s more exciting, but it’s also more dangerous.
Ultimately, the best way to use git pull and git push depends on your needs. If you need to keep your local repository up-to-date with the latest changes, then you should use git pull. If you need to share your changes with others, then you should use git push.
Here is a joke about git pull and git push:
Why did the Git developer cross the road?
To fetch the latest changes.
User Experience Meme
Bad user experience (UX) happens when you start with high hopes, but then things start to go wrong. The website is slow, the buttons are hard to find, and the error messages are confusing. By the end of the experience, you’re just hoping to get out of there as soon as possible.
Here are some examples of bad UX:
- A website that takes forever to load.
- A form that asks for too much information.
- An error message that doesn’t tell you what went wrong.
- A website that’s not mobile-friendly.
Bad UX can be frustrating and even lead to users abandoning a website or app altogether. So, if you’re designing a user interface, make sure to put the user first and create an experience that’s easy and enjoyable to use.
5. Open AI Memes and Jokes
OpenAI is a non-profit research company that is working to ensure that artificial general intelligence benefits all of humanity. They have developed a number of AI tools that are already making our lives easier, such as:
- GPT-3: A large language model that can generate text, translate languages, write different kinds of creative content, and answer your questions in an informative way.
- Dactyl: A robot hand that can learn to perform complex tasks by watching humans do them.
- Five: A conversational AI that can help you with tasks like booking appointments, making reservations, and finding information.
OpenAI’s work is also leading to the obsolescence of some traditional ways of work. For example, GPT-3 is already being used by some businesses to generate marketing copy, and it is likely that this technology will eventually replace human copywriters altogether.
Here is a light and funny way to think about the impact of OpenAI on our lives:
- OpenAI is like a genie in a bottle. It can grant us our wishes, but it’s up to us to use its power wisely.
- OpenAI is like a new tool in the toolbox. It can help us do things that we couldn’t do before, but it’s not going to replace us.
- OpenAI is like a new frontier. It’s full of possibilities, but it’s also full of risks.
Ultimately, the impact of OpenAI on our lives is still unknown. But one thing is for sure: it’s going to change the world in ways that we can’t even imagine.
Here is a joke about OpenAI:
What do you call a group of OpenAI researchers?
A think tank.
In addition to being fun, memes and jokes can also be a great way to discuss complex topics in a more accessible way. For example, a meme about the difference between supervised and unsupervised learning can help people who are new to these topics understand the concepts more visually.
Of course, memes and jokes are not a substitute for serious study. But they can be a fun and engaging way to learn about data science, machine learning, artificial intelligence, and statistics.
So next time you’re looking for a laugh, be sure to check out some memes and jokes about data science. You might just learn something!